XGBoost with GPUs and Multi-core CPU
We run XGBoost on a multi-core CPU and GPUs. On the CPU, the speed is maximum with 16 cores and does not improve with more cores. A speed-up of 29% can be obtained with a single GPU than the CPU with 16 cores. Interestingly, we do not observe speed-up from one GPU to two GPUs.
Data
The data used for the regression have 270230 rows, 77 predictors, and one predicted parameter.
Multi-core CPU
The CPU is an Intel Xeon W-2295 processor with a 3.00GHz base processor frequency and 36 cores.
The n_jobs
argument can set the number of cores in the CPU in XGBRegressor
in Python:
import xgboost as xgb
for n in [1, 2, 4, 8, 16, 20, 24, 28, 32]:
start = time.time()
reg = xgb.XGBRegressor(verbosity=0,
tree_method='hist',
n_estimators=1000,
n_jobs=n)
reg.fit(X, y) # X shape=(270230,77), y shape =(230230,)
end = time.time()
print(f'n_cores={n}; time={end-start:.2f}s')
The computation time of the regression as a function of the number of CPU cores is shown in Figure 1. The time is the shortest with 16 cores and remains more or less the same for more cores.
I suspected that the optimal number of cores might depend on the workload. But using a much larger dataset with 1,000,000 rows and 200 predictors, the computation time is still the shortest with 16 cores.
GPU
The computer has two NVIDIA Quadro RTX 6000 GPUs.
Single GPU
Running on a single GPU is straightforward by setting tree_method
to gpu_hist
.
xgb.XGBRegressor(tree_method='gpu_hist', n_estimators=ntrees)
Then we varied the workload by varying the number of trees in the XGBoost regressor and compared the CPU and GPU computation time.
As shown in Figure 2, the relationship between CPU and GPU computation time is linear:
\[\mathrm{GPU\ Time} = \mathrm{slope}\times\mathrm{CPU\ Time}.\]The slope reflects the relative speed. Its value is less than 1, meaning the GPU is faster than the CPU. For example, the GPU is 29% faster than the CPU with 16 cores.
Number of CPU Cores | Slope | Speed-up |
---|---|---|
4 | 0.34 | 66% |
8 | 0.55 | 45% |
16 | 0.71 | 29% |
Dual GPUs
As shown in the preceding section, we used the sklearn API to run on a single GPU. There is no obvious way to run on multiple GPUs by using the sklearn API alone. Dask-CUDA is needed to create a GPU cluster to run on multiple GPUs.
import xgboost as xgb
import dask.array as da
from dask.distributed import Client
from dask_cuda import LocalCUDACluster
n_chunks = 8 #number of chunks
chunksize = int(X.shape[0]/n_chunks)
n_trees = 20000 # number of tree estimators
n_gpus = 2 # number of GPUs
if __name__ == "__main__":
with LocalCUDACluster(n_workers=n_gpus) as cluster:
with Client(cluster) as client:
start = time.time()
xx = da.from_array(X.to_numpy(), chunks=chunksize)
yy = da.from_array(y.to_numpy().reshape(-1,1),
chunks=chunksize)
end1 = time.time()
clf = xgb.dask.DaskXGBRegressor(n_estimators=n_trees,
tree_method="gpu_hist")
clf.client = client
clf.fit(xx,yy)
end = time.time()
print(f'data loading={end1-start:.2f}; computation={end-end1:.2f}')
A couple of settings are essential for multiple GPUs:
n_workers
inLocalCUDACluster
can be set to 1 to use a single GPU and more than 1 for multiple GPUs.- The data must be converted into Dask arrays and broken into multiple chunks. If there is only a single chunk in the data, XGBoost will run on only one GPU even though
n_workers
is set to more than 1 inLocalCUDACluster
.
The GPU status can be monitored with the nvidia-smi
command, and Figures 3 and 4 show the status of running on a single GPU and two GPUs, respectively.
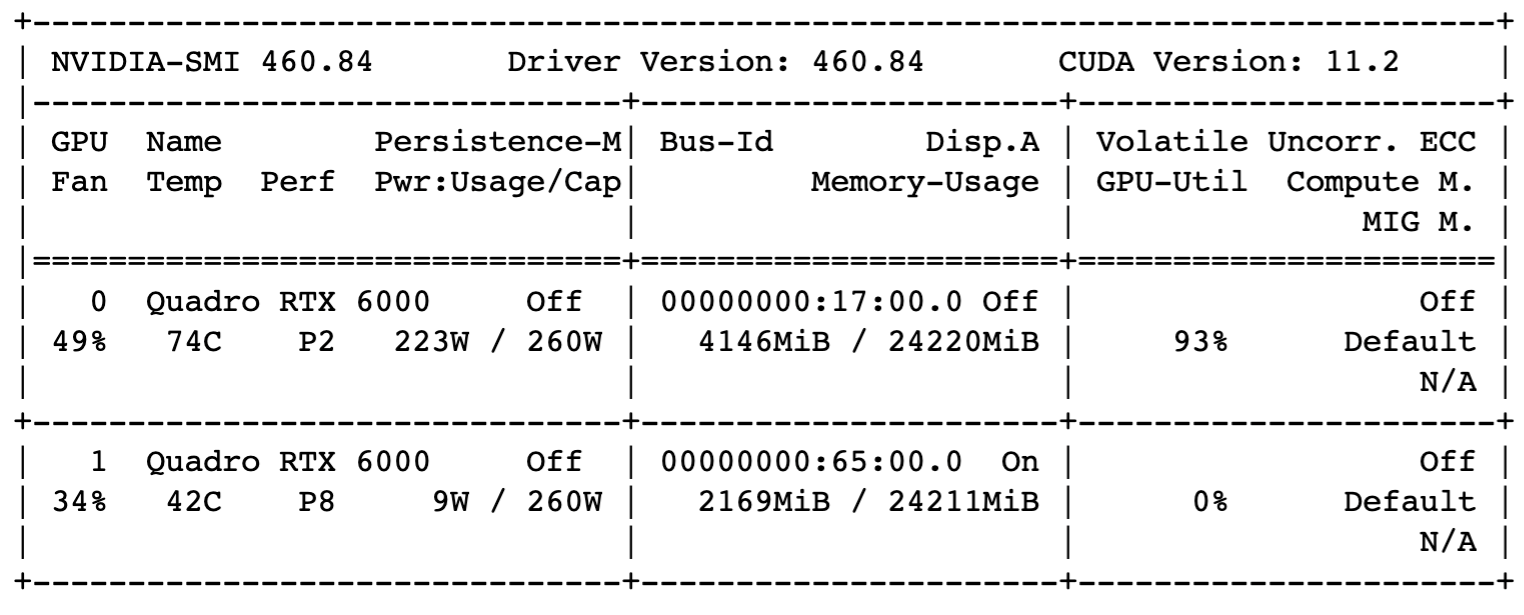
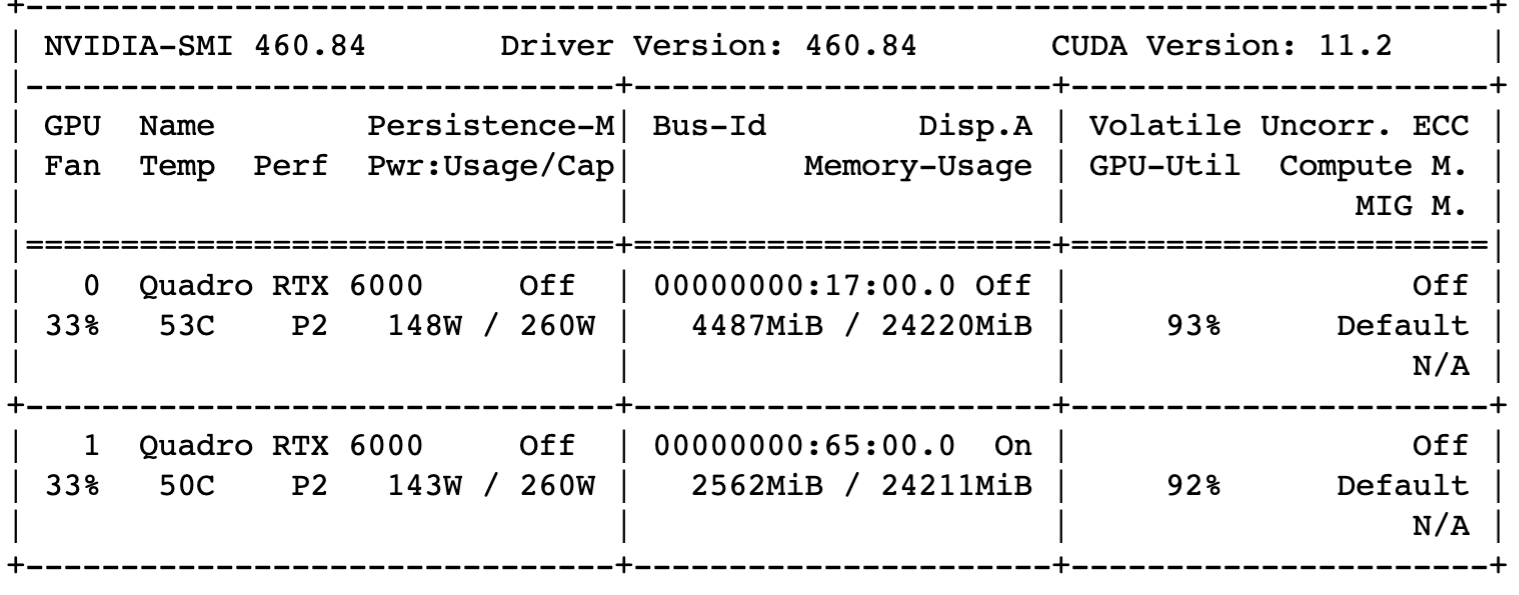
The computation time on a single GPU and two GPUs is almost the same, as shown in the table below. There is no speed-up with more GPUs.
Number of GPUs | n_trees | # of Chunks | Dask Array Loading Time (s) | Computation Time (s) |
---|---|---|---|---|
1 | 20000 | 8 | 1.15 | 93.7 |
2 | 20000 | 8 | 1.35 | 91.8 |
2 | 20000 | 20 | 1.54 | 91.4 |
Summary
We have evaluated two ways to speed up XGBoost: multiple CPU cores and GPUs.
On the computer used for this study, the speed-up on the 36-core CPU is maximized with 16 cores. Using more than 16 cores does not improve performance further.
A single GPU improves XGBoost speed by 29% from the best CPU performance with 16 cores. Dask-CUDA is needed to run XGBoost on multiple GPUs. In this study, however, two GPUs perform no better than a single GPU.